- Researchers have combined tagging technology, satellite data and machine learning to create a model that predicts the potential locations of unknown populations of reticulated giraffes in Africa.
- The model also predicts suitable habitats where giraffes could be moved to for conservation purposes.
- Reticulated giraffes are endangered, with their populations declining due to habitat loss triggered by deforestation, urbanization and agricultural expansion.
- Findings from the model have estimated huge swaths of suitable habitats in Kenya, Ethiopia and Somalia; they’ve also contributed to the translocation of 14 giraffes to Angola, from where the animals were driven out by conflict years back.
Stephanie Fennessy and her husband have been tagging giraffes in Africa since the early 2000s. The couple initially started out by using modified elephant collars. They later switched to using head harnesses, and then tags that could be fitted onto ossicones, the pair of horn-like structures at the top of a giraffe’s head. The latest iteration in this evolution comprises GPS tags that can be attached to ears or tails.
Over the years, as the tags have shrunk in size, the volume of data they’ve been able to gather has grown. Fennessy’s early tags could only track the movements of giraffes. Now, that information is being combined with satellite imagery and machine-learning tools to predict where giraffes might be, as well as to identify suitable habitats where the animals could be moved to for conservation purposes.
“It is helping us to find still remnant populations of giraffes that we don’t know about yet by just looking at what habitat they theoretically can thrive in,” Fennessy, executive director and co-founder of Namibia-based nonprofit the Giraffe Conservation Foundation, told Mongabay in a video interview. “It also helps us identify potential habitats and support a lot of translocations to bring giraffes back into their historical habitats.”
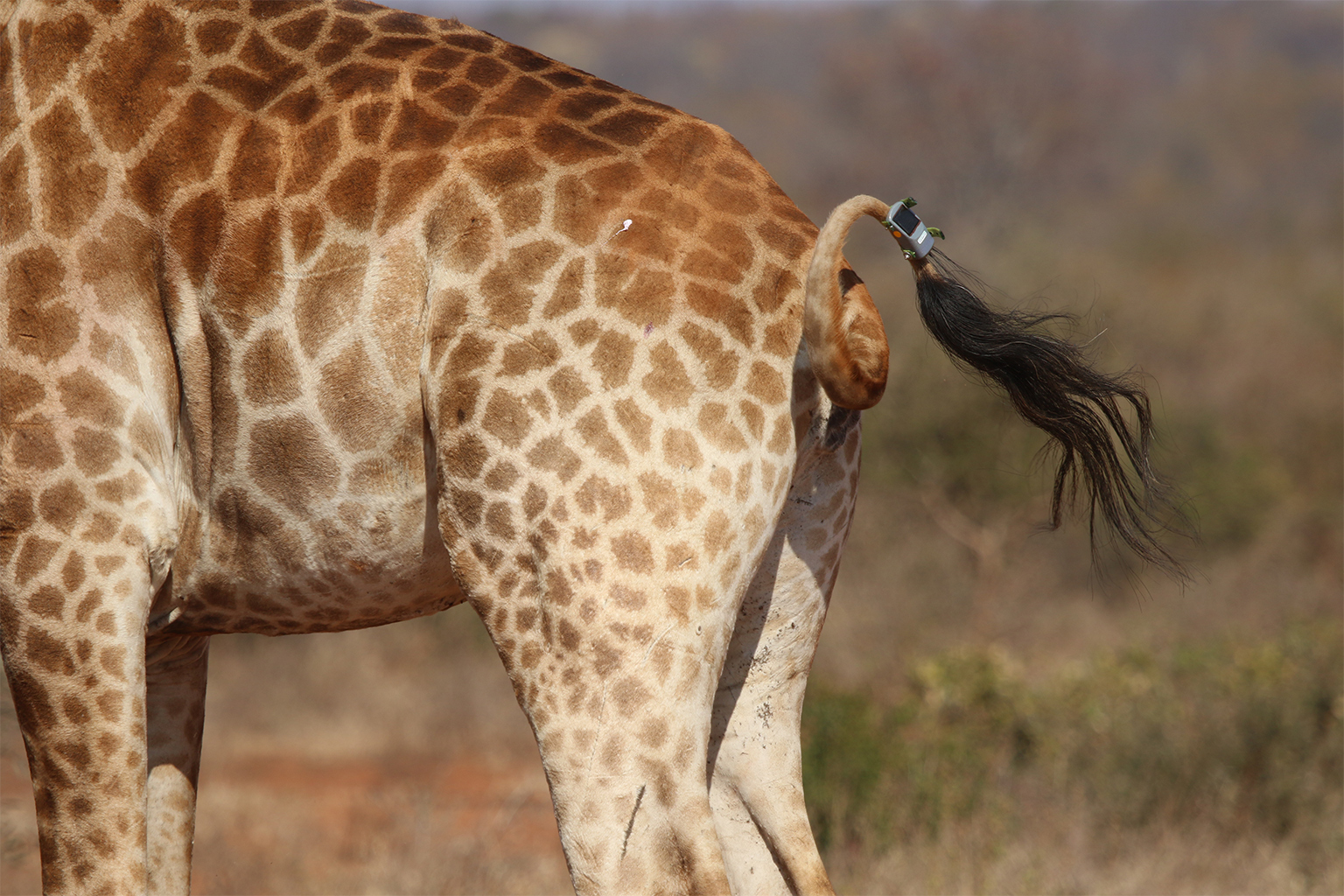
The Giraffe Conservation Foundation collaborated with other organizations, including the Smithsonian Conservation Biology Institute, to test out the feasibility of the technique to protect reticulated giraffes. A study done by the team, and published in the journal Animal Conservation, illustrates the possibility of combining “spatial resolution imagery with telemetry data to guide conservation programs of threatened terrestrial species.”
Reticulated giraffes (Giraffa reticulata) are an endangered species. They’re easily identified by their rich orange-brown coat interspersed with bright white lines crisscrossing the entire body. The animals are found mostly in Kenya, Ethiopia and Somalia. However, habitat loss triggered by deforestation, urbanization and agricultural expansion have led to a decline in their population over the years. According to estimates by the Giraffe Conservation Foundation, there are only approximately 16,000 individuals left in the wild.
Despite plummeting populations, however, giraffes as a whole are notoriously understudied. Fennessy said their protection often doesn’t feature on conservation organizations’ priority lists.
“For a long time, they really were a bit of a forgotten megafauna,” she said. “The biggest challenge is the lack of knowledge. Even seasoned conservationists are not aware that giraffes are in trouble.”
Studying giraffes isn’t easy. For one, Fennessy said, there might be remnant populations whose existence might be unknown to scientists. Since giraffes often move in very remote areas, it’s also hard to understand the characteristics of the habitats they prefer to roam around and live in.
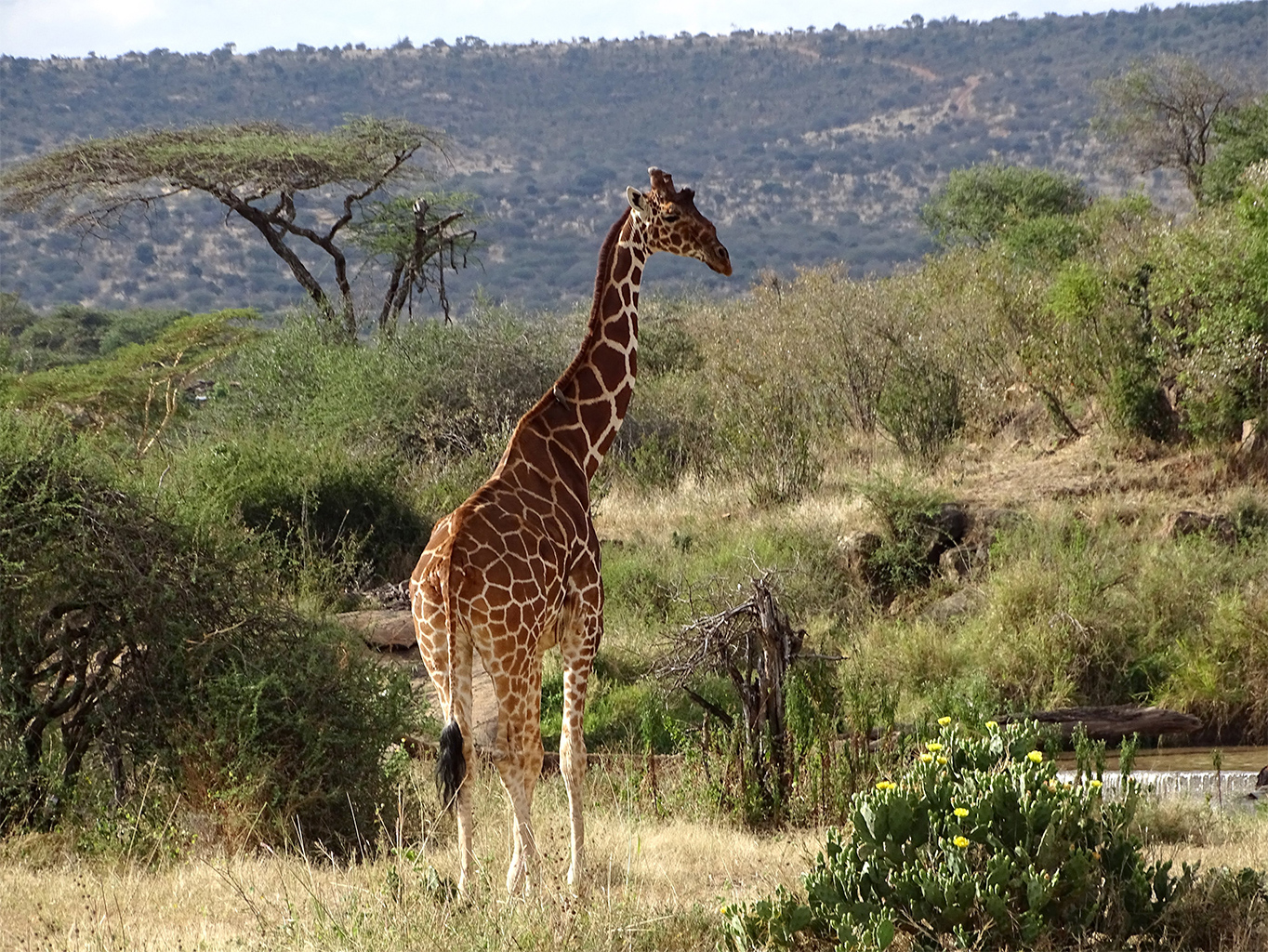
“Sometimes we don’t see them, and then we really don’t know where they go,” she said. “They also go into very mountainous areas where we can’t easily follow them.”
To protect them, it’s imperative to know where they are. And to move giraffes for conservation purposes, it’s essential to study existing populations and understand the characteristics of their habitats.
“To recover populations, conservation organizations translocate animals into new habitats because they want to avoid inbreeding and ensure genetic diversity,” Ramiro Crego, lead author of the recent study and a postdoctoral researcher at the Smithsonian Conservation Biology Institute’s Conservation Ecology Center, told Mongabay in a video interview.
To find a solution to the problem, Crego and his team developed a machine-learning model that predicts the locations of unknown populations as well as areas that are potentially suitable giraffe habitats.
As part of the study, 31 reticulated giraffes were fitted with solar-powered GPS tags in central and northern Kenya in 2019 and 2020. Once the team tracked where the giraffes were moving, they overlaid that data with satellite images to help them understand the environmental traits of the areas the giraffes inhabit, including vegetation, soil and surface ruggedness.
“We combined these high-resolution data, and got information on where these individuals are moving every hour,” Crego said. “We can zoom in and go down on the terrain and get information about what conditions the animals are using.”
They then fed the data into a machine-learning algorithm, which was trained on the attributes that the giraffes prefer in their surroundings. The model then got to work producing maps of areas that matched the factors that giraffes are likely attracted to.
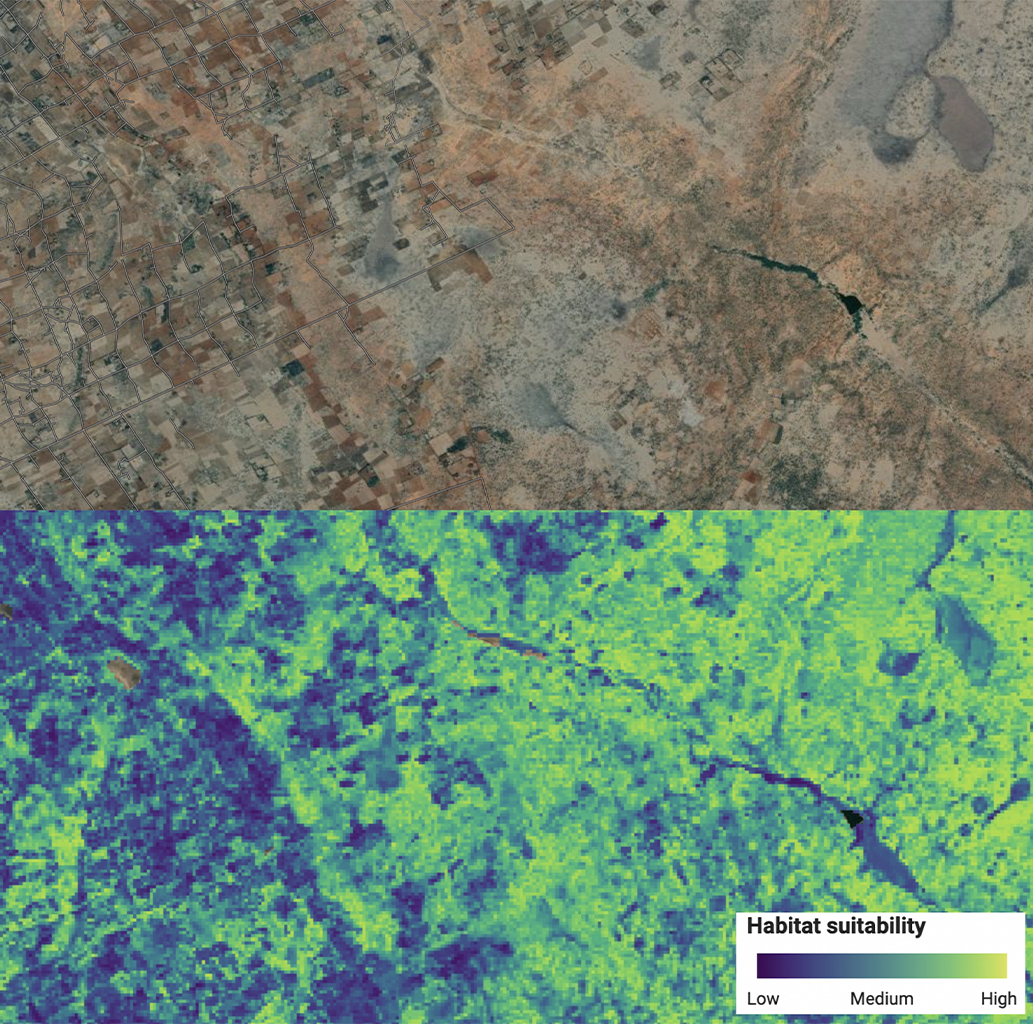
“It displays the probability of a giraffe using a particular area,” Crego said. “It will show that the giraffe might use this pixel here, or that pixel there, and you get a map with highlighted colors that shows areas with high and low probabilities.”
It’s not always perfect. The further a location moves away from the area that the model was trained on, the more uncertain the results will be. Additionally, Crego said, the usual caveats of machine-learning models apply: “When you’re doing analysis, there is never enough data. Even though you have a lot of data, you always need more to get better predictions.”
However, despite the gaps, the model has already been used for conservation on the ground. According to the study, the model has been able to predict “a total of 5,519 square kilometers of potentially suitable habitat in Kenya, 963 square kilometers in Ethiopia, and 147 square kilometers in Somalia.” That’s a total of 6,629 km2 (2,559 square miles), or 1% of Somalia’s landmass.
The findings have contributed to the development of Ethiopia’s Giraffe Conservation Action Plan. In July, the model also played a role in the translocation of 14 Angolan giraffes from Namibia to Iona National Park in Angola, where giraffes went extinct after they were driven out by civil unrest and the ensuing conflict. The team has also made the model publicly available in the form of a web app with the aim of helping wildlife managers make informed decisions about species reintroduction.
Both Fennessy and Crego said the framework delineated in the study — combining telemetry data with satellite imagery to predict potential habitats — could be adapted and used for other species in different locations across the world.
“The decision to move animals is sometimes more political than scientific. But this can be one layer of information that helps in those efforts,” Crego said. “To think that this model could actually be useful, it’s very gratifying to see.”
Banner image: The team at the Giraffe Conservation Foundation with a giraffe. Image courtesy of GCF.
Abhishyant Kidangoor is a staff writer at Mongabay.